Table Of Content
- Book traversal links for Lesson 5: Introduction to Factorial Designs
- Factorial experiment
- Sequential Procedure for Strategically Finding a Model
- What Are Factorial Experiments and Why Can They Be Helpful?
- Checking Residuals Using Minitab's Four in One Plot
- Coding Systems for the Factor Levels in the Factorial Design of Experiment
- Significance Level
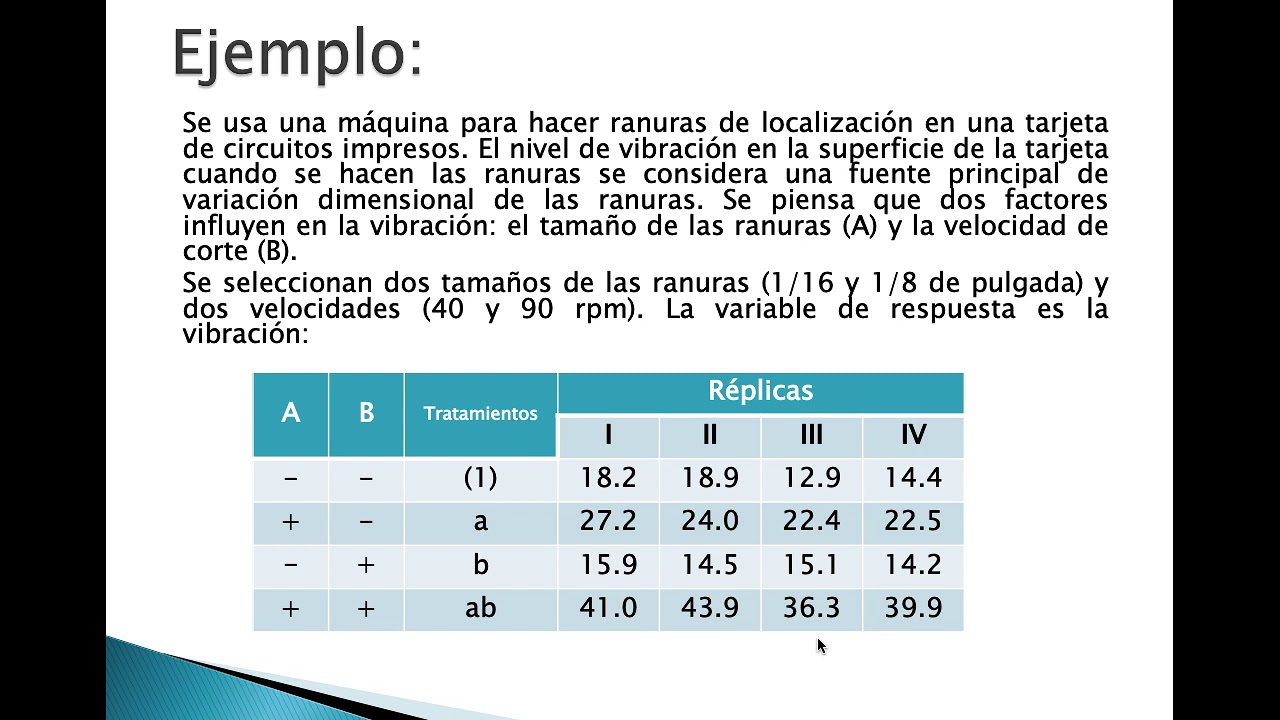
Multilevel modeling is used to analyze data that is nested within multiple levels, such as students nested within schools or employees nested within companies. SEM is a statistical technique used to model complex relationships between variables. ANOVA is a statistical technique used to compare means across two or more groups in order to determine whether there are significant differences between the groups. There are several types of ANOVA, including one-way ANOVA, two-way ANOVA, and repeated measures ANOVA. Inferential statistics are used to make inferences or generalizations about a larger population based on the data collected in the study.
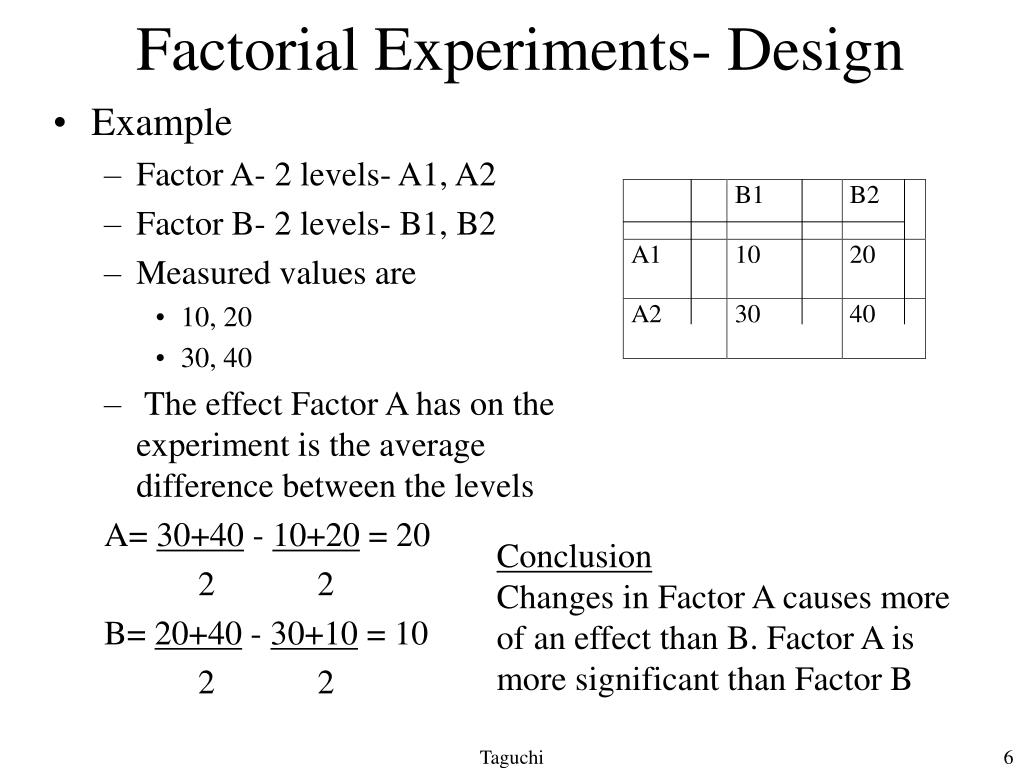
Book traversal links for Lesson 5: Introduction to Factorial Designs
In the example above the A, B and C each are defined by a contrast of the data observation totals. Therefore you can define the contrast AB as the product of the A and B contrasts, the contrast AC by the product of the A and C contrasts, and so forth. Introduction to Statistics is our premier online video course that teaches you all of the topics covered in introductory statistics. Statology Study is the ultimate online statistics study guide that helps you study and practice all of the core concepts taught in any elementary statistics course and makes your life so much easier as a student. In the previous plot, the two lines were roughly parallel so there is likely no interaction effect between watering frequency and sunlight exposure. Research findings are often presented to readers using graphs or tables.
Factorial experiment
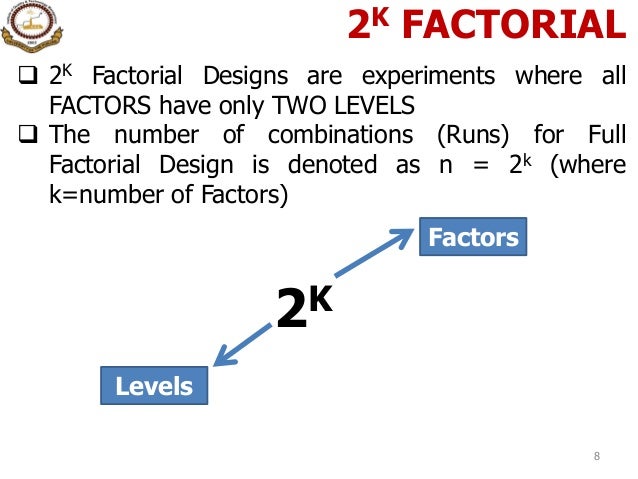
While simple psychology experiments look at how one independent variable affects one dependent variable, researchers often want to know more about the effects of multiple independent variables. Ambitious, multifactor, factorial experiments designed to evaluate clinical ICs can and do work for the purpose of intervention component screening (Baker et al., 2016; Collins et al., 2016; Collins, Murphy, & Strecher, 2007; Fraser et al., 2014). We believe that their potential to yield unique data, and to do so efficiently, should make factorial screening experiments a core strategy in the process of developing effective treatments (Collins et al., 2016). For instance, not only do such designs permit the screening of multiple intervention components in a single experiment, but compared with RCT designs, factorial experiments permit more precise estimates of mediational effects.
Sequential Procedure for Strategically Finding a Model
Experimental based assessment of formwork pressure theoretical design models for self-compacting concrete - ScienceDirect.com
Experimental based assessment of formwork pressure theoretical design models for self-compacting concrete.
Posted: Thu, 01 Jun 2023 07:00:00 GMT [source]
All rights are reserved, including those for text and data mining, AI training, and similar technologies. For all open access content, the Creative Commons licensing terms apply. Once the terms have been chosen, the next step is determining which graphs should be created. The types of graphs can be selected by clicking on "Graphs..." in the main "Analyze Factorial Design" menu. Additional modifications to the design include randomizing and renumbering the design. These are very straightforward modifications which affect the ordering of the trials.
The Main Total Effect can be related to input variables by moving along the row and looking at the first column. If the row in the first column is a2b1c1 then the main total effect is A. To get a mean factorial effect, the totals needs to be divided by 2 times the number of replicates, where a replicate is a repeated experiment.
Checking Residuals Using Minitab's Four in One Plot
Replication involves conducting the same experiment with different samples or under different conditions to increase the reliability and validity of the results. Field experiments are conducted in naturalistic settings and allow for more realistic observations. However, because field experiments are not as controlled as laboratory experiments, they may be subject to more sources of error.
Coding Systems for the Factor Levels in the Factorial Design of Experiment
Of course, there is increased efficiency as investigators can screen more components at a reduced expenditure of resources. In an RCT an “active” treatment arm or condition is statistically contrasted with a “control” treatment arm or condition (Friedman, Furberg, & Demets, 2010). The two conditions should be identical except that the control condition lacks one or more ICs or features that are provided to the active condition. The random assignment of participants to the treatment arms means that the two groups of assigned participants should differ systematically only with regard to exposure to those features that are intentionally withheld from the controls.
Significance Level
If more than one outcome variable is used in analyses, the number of models computed and effects tested grow quickly. Various approaches have been suggested for dealing with the challenge posed by so many statistical comparisons being afforded by complex factorial designs (Couper et al., 2005; Green, Liu, & O’Sullivan, 2002). As opposed to an RCT, where the focus is on demonstrating effects that are highly unlikely to be due to chance, the screening experiment is focused on relative promise of the tested ICs. In addition, the use of a large number of factors allows for built-in evaluations of the robustness of the main effects of the ICs. This is because, as noted earlier, such effects are determined by averaging over the other component effects (with effect coding). A factorial design is a type of experiment that involves manipulating two or more variables.
In a three-factor experiment, this issue can be addressed by replication, but for larger studies this might be infeasible owing to the large number of treatments. Higher order interactions can reflect complex patterns that defy easy interpretation. However, they also reveal information that is unique and of potentially great value. Further, this problem is reduced if factorial designs are used as screening experiments, whose purpose is not to identify the single best combination of ICs (Collins et al., 2009). Rather such experiments are used to identify the ICs that are amongst the best.
The second way of looking at the interaction is to start by looking at the other variable. For example, does the effect of time since last meal depend on the levels of the tired variable? Look first at the effect of time since last meal only for the red bars in the “not tired” condition. The red bar in the 1 hour condition is 1 unit smaller than the red bar in the 5 hour condition. Next, look at the effect of time since last meal only for the green bars in the “tired” condition. The green bar in the 1 hour condition is 3 units smaller than the green bar in the 5 hour condition.
In a within-subjects factorial design, all of the independent variables are manipulated within subjects. All participants could be tested both while using a cell phone and while not using a cell phone and both during the day and during the night. This would mean that each participant would need to be tested in all four conditions. The advantages and disadvantages of these two approaches are the same as those discussed in Chapter 5.
We have already seen that factorial experiments can include manipulated independent variables or a combination of manipulated and non-manipulated independent variables. But factorial designs can also consist exclusively of non-manipulated independent variables, in which case they are no longer experiments but correlational studies. Consider a hypothetical study in which a researcher measures two variables. The research then also measure participants’ willingness to have unprotected sexual intercourse. This study can be conceptualized as a 2 x 2 factorial design with mood (positive vs. negative) and self-esteem (high vs. low) as between-subjects factors. This design can be represented in a factorial design table and the results in a bar graph of the sort we have already seen.
Video recording involves recording participants’ behavior or interactions using cameras or other recording equipment. This method can be used to capture detailed information about participants’ behavior or to analyze social interactions. Behavioral measures involve measuring participants’ behavior directly, such as through reaction time tasks or performance tests.
In this design, each participant is exposed to all of the different treatments or conditions, either in a random order or in a predetermined order. In this design, participants are randomly assigned to one of two or more groups, and each group is exposed to a different treatment or condition. Experimental design is a process of planning and conducting scientific experiments to investigate a hypothesis or research question. It involves carefully designing an experiment that can test the hypothesis, and controlling for other variables that may influence the results. The first is to take a higher order interaction out of the model and use them as the estimate of error.
Is the adherence intervention different enough in its various forms (across medications) so that it no longer constitutes a single, coherent component? If that is true, its effects cannot be interpreted in a straightforward manner. If no adherence main effect is found, is that because this component was inconsistently delivered (adjusted for each medication)? In sum, investigators should be cognizant of the possible effects of such intervention adjustment and consider options for addressing them (e.g., by making only essential adjustments to a component, nesting an adjusted factor in the design). Thus, investigators must decide if they wish to directly compare two treatment conditions (and these may be multicomponential) with one another, without the results being affected by the presence of other experimental factors being manipulated.
For example, Schnall and her colleagues were justified in concluding that disgust affected the harshness of their participants’ moral judgments because they manipulated that variable and randomly assigned participants to the clean or messy room. But they would not have been justified in concluding that participants’ private body consciousness affected the harshness of their participants’ moral judgments because they did not manipulate that variable. It could be, for example, that having a strict moral code and a heightened awareness of one’s body are both caused by some third variable (e.g., neuroticism). Thus it is important to be aware of which variables in a study are manipulated and which are not.
No comments:
Post a Comment